the 1987 edition of The New Palgrave, edited by Eatwell, Milgate & Newman.
Herbert A Simon寫了幾條:
Behaviour Economics
Bounded Rationality
Causality in Economic Model
Evans, Griffith Conrad, 1887-1973
Satisficing
*****http://www.dictionaryofeconomics.com/dictionary
Search results
The results of your search are shown below. If you prefer, you may use the filter options to refine the list further, or search again.
Your search for "herbert simon" over the entire article content within the 2008, 2009, 2010, 2011, 2012 and 2013 editions returned 26 results.
Articles on topic:
All current edition articles
Result page: 1 2 3 Next >
1. Simon, Herbert A. (1916–2001)
This article discusses how Simon's vision for behavioural economics (and social science generally) was found in the context of his early work in public ...
By Mie Augier. From The New Palgrave Dictionary of Economics, Second Edition, 2008
2. Ando, Albert K. (1929–2002)
Albert K. Ando was an eminent Japanese-born American economist who made many seminal contributions in a broad range of areas of economics. Born in Tokyo, ...
By Charles Yuji Horioka. From The New Palgrave Dictionary of Economics, Second Edition, 2008
3. superstars, economics of
Gigantic incomes and rare talents attract attention and elicit a search for an explanation. Sherwin Rosen has provided us with an elegant neoclassical ...
By Walter Y. Oi. From The New Palgrave Dictionary of Economics, Second Edition, 2008
4. rationality, bounded
‘Bounded rationality’ refers to rational choice that takes into account the cognitive limitations of the decision-maker – limitations of both knowledge ...
By Herbert A. Simon. From The New Palgrave Dictionary of Economics, Second Edition, 2008
5. conventionalism
Conventionalism is the methodological doctrine that asserts that explanatory ideas should not be considered true or false but merely better or worse. ...
By Lawrence A. Boland. From The New Palgrave Dictionary of Economics, Second Edition, 2008
6. Sargent, Thomas J. (born 1943)
Thomas J. Sargent is the 2011 recipient of the Nobel Prize in Economic Sciences (along with Christopher Sims). Sargent has been instrumental in the development ...
By Esther-Mirjam Sent. From The New Palgrave Dictionary of Economics, Online Edition, 2012
7. Granger–Sims causality
The concept of Granger–Sims causality is discussed in its historical context. There follows a review of the subsequent literature that explored conditions ...
By G. M. Kuersteiner. From The New Palgrave Dictionary of Economics, Second Edition, 2008
8. causality in economics and econometrics
Economics was conceived as early as the classical period as a science of causes. The philosopher–economists David Hume and J. S. Mill developed the conceptions ...
By Kevin D. Hoover. From The New Palgrave Dictionary of Economics, Second Edition, 2008
9. Selten, Reinhard (born 1930)
This article describes the main contributions to game theory and boundedly rational economic behaviour of Reinhard Selten, winner, together with John ...
By Eric van Damme. From The New Palgrave Dictionary of Economics, Second Edition, 2008
10. competition and selection
The claim that a business firm must maximize profit if it is to survive serves as an informal statement of the common conclusion of a class of theorems ...
By Sidney G. Winter. From The New Palgrave Dictionary of Economics, Second Edition, 2008
11. experimental economics, history of
Contemporary experimental economics was born in the 1950s from the combination of the experimental method used in psychology and new developments in economic ...
By Francesco Guala. From The New Palgrave Dictionary of Economics, Second Edition, 2008
12. rationality, history of the concept
This article offers a historical and methodological perspective on the concept of rationality. It gives an overview of the various interpretations of ...
By Esther-Mirjam Sent. From The New Palgrave Dictionary of Economics, Second Edition, 2008
13. efficient markets hypothesis
The efficient markets hypothesis (EMH) maintains that market prices fully reflect all available information. Developed independently by Paul A. Samuelson ...
By Andrew W. Lo. From The New Palgrave Dictionary of Economics, Second Edition, 2008
14. Williamson, Oliver. E (born 1932)
Oliver E. Williamson is the 2009 co-recipient (with Elinor Ostrom) of the Nobel Memorial Prize in Economics, awarded ‘for his ...
By Scott E. Masten. From The New Palgrave Dictionary of Economics, Online Edition, 2010
15. satisficing
‘Satisficing’ (choosing an option that meets or exceeds specified criteria but is not necessarily either unique or the best) is an alternative conception ...
By Herbert A. Simon. From The New Palgrave Dictionary of Economics, Second Edition, 2008
16. Evans, Griffith Conrad (1887–1973)
A distinguished American mathematician and pioneer mathematical economist, Evans was born on 11 May 1887 in Boston, Massachusetts. Educated in mathematics ...
By Herbert A. Simon. From The New Palgrave Dictionary of Economics, Second Edition, 2008
17. firm, theory of the
It is doubtful if there is yet general agreement among economists on the subject matter designated by the title ‘theory of the firm’, on, that is, the ...
By G.C. Archibald. From The New Palgrave Dictionary of Economics, Second Edition, 2008
18. rational behaviour
A clear distinction must be drawn between (a) the type of behaviour that might be described as rational, and (b) rational behaviour models that might ...
By Amartya Sen. From The New Palgrave Dictionary of Economics, Second Edition, 2008
19. United States, economics in (1885–1945)
The history of American economics following the founding of the American Economic Association in 1885 is not a simple linear narrative of the triumph ...
By Bradley W. Bateman. From The New Palgrave Dictionary of Economics, Second Edition, 2008
20. input–output analysis
Input–output analysis is a practical extension of the classical theory of general interdependence which views the whole economy of a region, a country ...
By Wassily Leontief. From The New Palgrave Dictionary of Economics, Second Edition, 2008
21. power
We consider the exercise of power in competitive markets for goods, labour and credit. We offer a definition of power and show that if contracts are incomplete ...
By Samuel Bowles and Herbert Gintis. From The New Palgrave Dictionary of Economics, Second Edition, 2008
22. altruism, history of the concept
This article describes the incorporation from the early 1960s of seemingly unselfish behaviour into economics. Faced with the problem of accounting for ...
By Philippe Fontaine. From The New Palgrave Dictionary of Economics, Second Edition, 2008
23. models
Philosophical analysis of the historical development of modelling, as well as the programmatic statements of the founders of modelling, support three ...
By Mary S. Morgan. From The New Palgrave Dictionary of Economics, Second Edition, 2008
24. United States, economics in (1945 to present)
After 1945, American economics was transformed as radically as in the previous half century. Economists’ involvement in the war effort compounded changes ...
By Roger E. Backhouse. From The New Palgrave Dictionary of Economics, Second Edition, 2008
25. Modigliani, Franco (1918–2003)
This article focuses on the scholarly contributions of Franco Modigliani, 1985 Nobel laureate in economics. Particular attention is given to his formulation ...
By Richard Sutch. From The New Palgrave Dictionary of Economics, Second Edition, 2008
26. Fisher, Irving (1867–1947)
Irving Fisher was born in Saugerties, New York, on 27 February 1867; he was residing in New Haven, Connecticut at the time of his death in a New York ...
By James Tobin. From The New Palgrave Dictionary of Economics, Second Edition, 2008
*****
Your search for "satisficing" over the entire article content within the 2008, 2009, 2010, 2011, 2012 and 2013 editions returned 12 results.
Articles on topic:
All current edition articles
Result page: 1 2 Next >
1. satisficing
‘Satisficing’ (choosing an option that meets or exceeds specified criteria but is not necessarily either unique or the best) is an alternative conception ...
By Herbert A. Simon. From The New Palgrave Dictionary of Economics, Second Edition, 2008
2. rational behaviour
A clear distinction must be drawn between (a) the type of behaviour that might be described as rational, and (b) rational behaviour models that might ...
By Amartya Sen. From The New Palgrave Dictionary of Economics, Second Edition, 2008
3. rationality, bounded
‘Bounded rationality’ refers to rational choice that takes into account the cognitive limitations of the decision-maker – limitations of both knowledge ...
By Herbert A. Simon. From The New Palgrave Dictionary of Economics, Second Edition, 2008
4. Simon, Herbert A. (1916–2001)
This article discusses how Simon's vision for behavioural economics (and social science generally) was found in the context of his early work in public ...
By Mie Augier. From The New Palgrave Dictionary of Economics, Second Edition, 2008
5. economic man
Economic man ‘knows the price of everything and the value of nothing’, so said because he or she calculates and then acts so as to satisfy ...
By Shaun Hargreaves-Heap. From The New Palgrave Dictionary of Economics, Second Edition, 2008
6. competition and selection
The claim that a business firm must maximize profit if it is to survive serves as an informal statement of the common conclusion of a class of theorems ...
By Sidney G. Winter. From The New Palgrave Dictionary of Economics, Second Edition, 2008
7. efficient markets hypothesis
The efficient markets hypothesis (EMH) maintains that market prices fully reflect all available information. Developed independently by Paul A. Samuelson ...
By Andrew W. Lo. From The New Palgrave Dictionary of Economics, Second Edition, 2008
8. rationality, history of the concept
This article offers a historical and methodological perspective on the concept of rationality. It gives an overview of the various interpretations of ...
By Esther-Mirjam Sent. From The New Palgrave Dictionary of Economics, Second Edition, 2008
9. case-based decision theory
Case-based decision theory was developed by Gilboa and Schmeidler. This article describes the framework and lays out the axiomatic foundations of the ...
By Ani Guerdjikova. From The New Palgrave Dictionary of Economics, Online Edition, 2009
10. market competition and selection
There is a long history in economics of using market selection arguments in defence of rationality hypotheses. According to these arguments, rational ...
By Lawrence E. Blume and David Easley. From The New Palgrave Dictionary of Economics, Second Edition, 2008
11. egalitarianism
This article surveys a variety of egalitarian theories. We look at a series of different answers to the question of what the metric of justice should ...
By Harry Brighouse and Adam Swift. From The New Palgrave Dictionary of Economics, Second Edition, 2008
12. profit and profit theory
A theory of profit should address itself to at least three questions – about the size (volume) of profit, its share in total income and about the rate ...
By Meghnad Desai. From The New Palgrave Dictionary of Economics, Second Edition, 2008
2013年12月20日 星期五
2013年10月17日 星期四
Herbert A. Simon Dies at 84; Won a Nobel for Economics
Herbert A. Simon Dies at 84; Won a Nobel for Economics
By PAUL LEWIS
Published: February 10, 2001
Herbert A. Simon, an American polymath who won the
Nobel in economics in 1978 with a new theory of decision making and who
helped pioneer the idea that computers can exhibit artificial
intelligence that mirrors human thinking, died yesterday. He was 84.
He died at the Presbyterian University Hospital of
Pittsburgh, according to an announcement by Carnegie Mellon University,
which said the cause was complications after surgery last month. Mr.
Simon was the Richard King Mellon University Professor of Computer
Science and Psychology at the university -- a title that underscored the
breadth of his interests and learning.
Mr. Simon also won the A. M. Turing Award for his
work on computer science in 1975 and the National Medal of Science in
1986. In 1993, he was awarded the American Psychological Association's
award for outstanding lifetime contributions to psychology.
In 1994, he became one of only 14 foreign scientists
ever to be inducted into the Chinese Academy of Sciences and in 1995
was given awards by the International Joint Conferences on Artificial
Intelligence and the American Society of Public Administration.
Awarding him the Nobel, the Swedish Academy of
Sciences cited ''his pioneering research into the decision-making
process within economic organizations'' and acknowledged that ''modern
business economics and administrative research are largely based on
Simon's ideas.''
Professor Simon challenged the classical economic
theory that economic behavior was essentially rational behavior in which
decisions were made on the basis of all available information with a
view to securing the optimum result possible for each decision maker.
Instead, Professor Simon contended that in today's
complex world individuals cannot possibly process or even obtain all the
information they need to make fully rational decisions. Rather, they
try to make decisions that are good enough and that represent reasonable
or acceptable outcomes.
He called this less ambitious view of human decision
making ''bounded rationality'' or ''intended rational behavior'' and
described the results it brought as ''satisficing.''
In his book ''Administrative Behavior'' he set out
the implications of this approach, rejecting the notion of an omniscient
''economic man'' capable of making decisions that bring the greatest
benefit possible and substituting instead the idea of ''administrative
man'' who ''satisfices -- looks for a course of action that is
satisfactory or 'good enough.' ''
Professor Simon's interest in decision making led
him logically into the fields of computer science, psychology and
political science. His belief that human decisions were made within
clear constraints seemed to conform with the way that computers are
programmed to resolve problems with defined parameters.
In the mid-1950's, he teamed up with Allen Newell of
the Rand Corporation to study human decision making by trying to
simulate it on computers, using a strategy he called thinking aloud.
People were asked for the general reasoning
processes they went through as they solved logical problems and these
were then converted into computer programs that Professor Simon and Mr.
Newell thought equipped these machines with a kind of artificial
intelligence that enabled them to simulate human thought rather than
just perform stereotyped procedures.
The breakthrough came in December 1955 when
Professor Simon and his colleague succeeded in writing a computer
program that could prove mathematical theorems taken from the Bertrand
Russell and Alfred North Whitehead classic on mathematical logic,
''Principia Mathematica.''
The following January, Professor Simon celebrated
this discovery by walking into a class and announcing to his students,
''Over the Christmas holiday, Al Newell and I invented a thinking
machine.''
A subsequent letter to Lord Russell explaining his
achievement elicited the reply: ''I am delighted to know that 'Principia
Mathematica' can now be done by machinery. I wish Whitehead and I had
known of this possibility before we wasted 10 years doing it by hand.''
But in a much-cited 1957 paper Professor Simon
seemed to allow his own enthusiasm for artificial intelligence to run
too far ahead of its more realistic possibilities. Within 10 years, he
predicted, ''a digital computer will be the world's chess champion
unless the rules bar it from competition,'' while within the ''visible
future,'' he said, ''machines that think, that learn and that create''
will be able to handle challenges ''coextensive with the range to which
the human mind has been applied.''
Sure enough, the I.B.M computer Deep Blue did
finally beat the world chess champion Gary Kasparov last year -- about
three decades after Mr. Simon had predicted the event would occur.
Because artificial intelligence has not grown as
quickly or as strongly as Professor Simon hoped, critics of his thinking
argue that there are limits to what computers can achieve and that what
they accomplish will always be a simulation of human thought, not
creative thinking itself. As a result, Professor Simon's achievements
have sparked a passionate and continuing debate about the differences
between people and thinking machines.
Born on June 15, 1916, the son of German immigrants,
in Milwaukee, Herbert A. Simon attended public school and entered the
University of Chicago in 1933 with the intention of bringing the same
rigorous methodology to the social sciences as existed in physics and
other ''hard'' sciences.
As an undergraduate his interest in decision making
was aroused when he made a field study of Milwaukee's recreation
department. After receiving his bachelor's degree in 1936 he became an
assistant to Clarence E. Ridley of the International City Managers
Association and then continued work on administrative techniques in the
Bureau of Public Administration of the University of California at
Berkeley.
In 1942, he moved to the Illinois Institute of
Technology and in 1943 received his doctorate from the University of
Chicago for a dissertation subsequently published in 1947 as
''Administrative Behavior: A Study of Decision-Making Processes in
Administrative Organizations.''
In 1937, he married Dorothea Pye, who survives him
along with three children, Katherine Simon Frank of Minneapolis; Peter
A. Simon of Bryan, Tex.; and Barbara M. Simon of Wilder, Vt.; six
grandchildren, three step-grandchildren; and five great-grandchildren.
A member of the faculty of Carnegie Mellon
University since 1949, Professor Simon played important roles in the
formation of several departments and schools including the Graduate
School of Industrial Administration, the School of Computer Science and
the College of Humanities and Social Sciences' psychology department.
He published 27 books, of which the best known today
are ''Models of Bounded Rationality'' (1997), ''Sciences of the
Artificial''(1996) and ''Administrative Behavior''(1997).
In 1991 he published his autobiography, ''Models of
My Life,'' and remarked then about his vision of that all-vanquishing
computer hunched over the chess boards of the world: ''I still feel good
about my prediction. Only the time frame was a bit short.'' And so it
was.
Photo: Herbert A. Simon (Ken Andreyo/Carnegie Mellon University)
2013年10月16日 星期三
Herbert A. Simon《我生活的種種模式》(The Models of My Life ) 漢譯本序言
Herbert A. Simon《我生活的種種模式》(The Models of My Life ) 漢譯本序言
「我生活的種種模式」(1999/03)
(The Models of My Life ) 漢譯本序言要旨
在此,我想對我的朋友和讀者重述一下孔夫子的名言:
「我生活的種種模式」(1999/03)
(The Models of My Life ) 漢譯本序言要旨
司馬賀(Herbert A. Simon )
我
很幸運,生活在現代電子計算機誕生並由此導致了人工智能領域形成的年代。我的自傳中有很多發生在那些令人激動的歲月中的故事。對我的自傳,我主要的希望
是:它能給正在考慮以科學研究為職業,或剛進入科學研究事業的年輕人,提供一些有關科學研究生涯的激動人心的畫面。當然,這些畫面也許附著許多久遠的舊時
代的色彩,而且就地域而言,它也離中國很遠。但是,一個科學家想要探究未知世界的迫切感,是不拘於任何時間,不特定於這個地球上的任何地方的。無論我們生
活在那個世紀、那塊土地上,我們都會對這種迫切感有所響應,都會因發現對人類有價值的新思想和新事物而感到歡欣和滿意。在此,我想對我的朋友和讀者重述一下孔夫子的名言:
三人行必有我師焉**。
- *該書簡體字版,由上海東方出版中心出版,本網站下月期將陸續有評介文章。
- **司馬賀一生與近一百位朋友合著論文及書籍,他在書中說:很多美妙的靈感都存在朋友的腦中,取之不盡。
2013年10月14日 星期一
Herbert Simon致Hanching Chung 1999.2
約1999.2 Herbert Simon 來信Hanching Chung
Dear Mr. Cheng:
I forgot to answer one of your questions in my last message -- where
to get more information about my publications. The address of my
home page on the WWW is:
http://www.psy.cmu.edu/psy/faculty/hsimon/hsimon.html
At the very end of the page you will find a cross-reference to
five other files that contain my complete bibliography, arranged
chronologically. On the home page you will also find a short list of
some recent publications, and similar lists on my other web pages
in Computer Science, Philosophy, and GSIA (our School of Business).
Sincerely yours,
Herbert A. Simon
2013年6月26日 星期三
米爾頓·弗里德曼 (鄒至莊)
在H. A. Simon的諾貝爾經濟學授獎的演講中曾大力批評M. F的方法論的矛盾.....
我的老師米爾頓·弗里德曼(一)
我在1955年赴美國芝加哥大學當研究生,並上弗里德曼的價格理論課。當弗里德曼進教室上他的第一課,他已給學生留下了深刻的印象,深於我以前任何 其他的老師。在第一課,他顯示了經濟理論能解釋現實的經濟現象。他的思維是尖銳的,並對別人的話他能立即作反應。在芝加哥,我也很幸運地能向其他著名的經 濟學家和統計學家學習,但弗里德曼對我的影響最大。這些老師,也影響了我後來其他的研究,這些研究不能在這一系列文章里討論。
當我寫我的論文《美國汽車的需求》時,阿諾德•哈伯格當我的導師,但論文的初稿到弗里德曼的研討班討論,從他那裡,我得到了非常有價值的意見。這班 裡也研討了弗里德曼和Mieselman的著名研究,比較使用貨幣供應M或用政府和其它的支出A能更好地解釋國民收入Y,作了Y與M的回歸和Y與A的回歸 來比較。發現用M的解釋比較好。作回歸時弗里德曼必須區分兩個M的定義,第一個只包括貨幣和活期存款,第二個加以定期存款。弗里德曼說:“讓我們把第一個 稱M1,把第二個稱M2”。這是我們稱M1和M2的開始。
弗 里德曼建議我使用永久收入或預期收入,而不是當期的收入來解釋汽車的需求。我發現他的永久收入變量能更好地解釋汽車總存量的需求,而當期的收入能更好地解 釋當年購買汽車的變量。原因是當年的採購包括儲蓄,而儲蓄受當年收入的影響。稍後我將討論永久性收入的用處。弗里德曼的預期收入的概念引導了經濟學中重要 的期望概念。他的預期概念,假定預期數量的年度變化等於前期的實現數量和前期的預期數量差異的一部分。就是說觀察了前期的實現數量和前期的預期數量有差異 時,應把預期數量部分的調整。
弗里德曼用國家的預期收入來解釋國家的消費量,這項研究被授予諾貝爾獎。以前的經濟學界發現,國家消費量增加的百分比少於國家當期收入增加的百分 比。如果是這樣的話,當國家收入繼續增加時國家消費總需求將不足帶動國家收入的繼續加,導致經濟不景氣,需要政府支出來解決。根據弗里德曼的消費理論,當 預期收入繼續增加時消費會同比例地增加,不致發生經濟不景氣。結論是自由市場經濟能夠持續增長,不需政府的乾預。
對耐用品的需求
弗里德曼認為許多假說都能解釋過去的數據,因此只有當一個假說或理論能被用來預測未來的數據我們才能對它有信心。這個概念影響我作美國汽車需求的繼 續研究。1958年,在我論文完成後,我的論文導師阿諾德•哈伯格決定出版一本由他指導關於耐用品需求的論文集。由於我的論文已經在1957年發表,我不 得不寫另一篇論文給他。我疑問我使用到1953年的數據來估計的需求方程是否能夠用來預測1954年至1957年的數據。我需要用一個統計學的檢驗方法來 解答。因此我發現了Chow檢驗來解答這個問題。
在我研究汽車的需求時,應用了加速原理。因為汽車總存量的需求,由收入決定。本年購買的新汽車,是總存量的變化,因此它的需求,是由收入的變化決 定。在這里收入比速度,收入的變化率,可以比加速。本年增加或本年購買的耐用品,是由收入的變化決定,這命為加速度原理。後來我用它來解釋很多其他耐用品 的需求,包括在美國,中國及台灣等地耐用消費品的需求。
註:本文僅代表作者本人觀點,作者近期出版了《鄒至莊論中國經濟》一書。
本文責任編輯 徐瑾 jin.xu@ftchinese.com
我的老師米爾頓·弗里德曼(一)
美國普林斯頓大學經濟學榮譽教授 鄒至莊 為英國《金融時報》中文網撰稿
在未來一系列的三篇文章中,我將首先描述我在美國芝加哥大學當弗里德曼學生的經驗,然後描述他一部分的經濟學研究和我後來同樣的研究。這些文章的主要目的是說明如何應用經濟理論來解釋和解決現實的經濟問題,這些文章不足以討論弗里德曼研究的許多其它議題。
弗里德曼作為我的教師和Chow檢驗的發現我在1955年赴美國芝加哥大學當研究生,並上弗里德曼的價格理論課。當弗里德曼進教室上他的第一課,他已給學生留下了深刻的印象,深於我以前任何 其他的老師。在第一課,他顯示了經濟理論能解釋現實的經濟現象。他的思維是尖銳的,並對別人的話他能立即作反應。在芝加哥,我也很幸運地能向其他著名的經 濟學家和統計學家學習,但弗里德曼對我的影響最大。這些老師,也影響了我後來其他的研究,這些研究不能在這一系列文章里討論。
當我寫我的論文《美國汽車的需求》時,阿諾德•哈伯格當我的導師,但論文的初稿到弗里德曼的研討班討論,從他那裡,我得到了非常有價值的意見。這班 裡也研討了弗里德曼和Mieselman的著名研究,比較使用貨幣供應M或用政府和其它的支出A能更好地解釋國民收入Y,作了Y與M的回歸和Y與A的回歸 來比較。發現用M的解釋比較好。作回歸時弗里德曼必須區分兩個M的定義,第一個只包括貨幣和活期存款,第二個加以定期存款。弗里德曼說:“讓我們把第一個 稱M1,把第二個稱M2”。這是我們稱M1和M2的開始。
弗 里德曼建議我使用永久收入或預期收入,而不是當期的收入來解釋汽車的需求。我發現他的永久收入變量能更好地解釋汽車總存量的需求,而當期的收入能更好地解 釋當年購買汽車的變量。原因是當年的採購包括儲蓄,而儲蓄受當年收入的影響。稍後我將討論永久性收入的用處。弗里德曼的預期收入的概念引導了經濟學中重要 的期望概念。他的預期概念,假定預期數量的年度變化等於前期的實現數量和前期的預期數量差異的一部分。就是說觀察了前期的實現數量和前期的預期數量有差異 時,應把預期數量部分的調整。
弗里德曼用國家的預期收入來解釋國家的消費量,這項研究被授予諾貝爾獎。以前的經濟學界發現,國家消費量增加的百分比少於國家當期收入增加的百分 比。如果是這樣的話,當國家收入繼續增加時國家消費總需求將不足帶動國家收入的繼續加,導致經濟不景氣,需要政府支出來解決。根據弗里德曼的消費理論,當 預期收入繼續增加時消費會同比例地增加,不致發生經濟不景氣。結論是自由市場經濟能夠持續增長,不需政府的乾預。
對耐用品的需求
弗里德曼認為許多假說都能解釋過去的數據,因此只有當一個假說或理論能被用來預測未來的數據我們才能對它有信心。這個概念影響我作美國汽車需求的繼 續研究。1958年,在我論文完成後,我的論文導師阿諾德•哈伯格決定出版一本由他指導關於耐用品需求的論文集。由於我的論文已經在1957年發表,我不 得不寫另一篇論文給他。我疑問我使用到1953年的數據來估計的需求方程是否能夠用來預測1954年至1957年的數據。我需要用一個統計學的檢驗方法來 解答。因此我發現了Chow檢驗來解答這個問題。
在我研究汽車的需求時,應用了加速原理。因為汽車總存量的需求,由收入決定。本年購買的新汽車,是總存量的變化,因此它的需求,是由收入的變化決 定。在這里收入比速度,收入的變化率,可以比加速。本年增加或本年購買的耐用品,是由收入的變化決定,這命為加速度原理。後來我用它來解釋很多其他耐用品 的需求,包括在美國,中國及台灣等地耐用消費品的需求。
註:本文僅代表作者本人觀點,作者近期出版了《鄒至莊論中國經濟》一書。
本文責任編輯 徐瑾 jin.xu@ftchinese.com
2013年6月15日 星期六
Intuition Pumps and Other Tools for Thinking. By Daniel Dennett.
水泵啟動式財政 Pump Priming 新帕尔格雷夫经济学大 词典专题索引
Daniel Dennett: Intuition Pumps and Other Tools for Thinking
Contemporary philosophy
Pump-primer
Tools for pondering imponderables

“THINKING is hard,” concedes Daniel Dennett. “Thinking about some problems is so hard that it can make your head ache just thinking about thinking about them.” Mr Dennett should know. A professor of philosophy at Tufts University, he has spent half a century pondering some of the knottiest problems around: the nature of meaning, the substance of minds and whether free will is possible. His latest book, “Intuition Pumps and Other Tools for Thinking”, is a précis of those 50 years, distilled into 77 readable and mostly bite-sized chapters.
“Intuition pumps” are what Mr Dennett calls thought experiments that aim to get at the nub of concepts. He has devised plenty himself over the years, and shares some of them. But the aim of this book is not merely to show how the pumps work, but to deploy them to help readers think through some of the most profound (and migraine-inducing) conundrums.
As an example, take the human mind. The time-honoured idea that the mind is essentially a little man, or homunculus, who sits in the brain doing clever things soon becomes problematic: who does all the clever things in the little man’s brain? But Mr Dennett offers a way out of this infinite regress. Instead of a little man, what if the brain was a hierarchical system?
This pump, which Mr Dennett calls a “cascade of homunculi”, was inspired by the field of artificial intelligence (AI). An AI programmer begins by taking a problem a computer is meant to solve and breaking it down into smaller tasks, to be dealt with by particular subsystems. These, in turn, are composed of sub-subsystems, and so on. Crucially, at each level down in the cascade the virtual homunculi become a bit less clever, to a point where all it needs to do is, say, pick the larger of two numbers. Such homuncular functionalism (as the approach is known in AI circles) replaces the infinite regress with a finite one that terminates at tasks so dull and simple that they can be done by machines.
Of course the AI system is designed from the top down, by an intelligent designer, to perform a specific task. But there is no reason why something similar couldn’t be built up from the bottom. Start with nerve cells. They are certainly not conscious, at least in any interesting sense, and so invulnerable to further regress. Yet like the mindless single-cell organisms from which they have evolved (and like the dullest task-accomplishing machines), each is able to secure the energy and raw materials it needs to survive in the competitive environment of the brain. The nerve cells that thrive do so because they “network more effectively, contribute to more influential trends at the [higher] levels where large-scale human purposes and urges are discernible”.
From this viewpoint, then, the human mind is not entirely unlike Deep Blue, the IBM computer that famously won a game of chess against Garry Kasparov, the world champion. The precise architecture of Mr Kasparov’s brain certainly differs from Deep Blue’s. But it is still “a massively parallel search engine that has built up, over time, an outstanding array of heuristic pruning techniques that keep it from wasting time on unlikely branches”.
Those who insist Deep Blue and Mr Kasparov’s mind must surely be substantially different will balk at this. They may well be right. But the burden of proof, Mr Dennett argues, is on them, for they are in effect claiming that the human mind is made up of “wonder tissue” with miraculous, mind-moulding properties that are, even in principle, beyond the reach of science—an old-fashioned homunculus in all but name.
Mr Dennett’s book is not a definitive solution to such mind-benders; it is philosophy in action. Like all good philosophy, it works by getting the reader to examine deeply held but unspoken beliefs about some of our most fundamental concerns, like personal autonomy. It is not an easy read: expect to pore over some passages more than once. But given the intellectual gratification Mr Dennett’s clear, witty and mercifully jargon-free prose affords, that is a feature, not a bug.
great Herbert Simon /Daniel Kahneman《 思考,快與慢》/ the priming effects 爭議
Some of the
evidence for this view is convincingly presented in Daniel Kahneman’s
recent book “Thinking Fast and Slow”: spectacular failures of expertise
include predictions of the future value of wine, the performance of
baseball players, the health of newborn babies and a couple’s prospects
for marital stability.
有关这一观点,丹尼尔·卡尼曼
(Daniel Kahneman)在其新近著述《思考:快与慢》(Thinking Fast and
Slow)中展示了一些令人信服的证据:就葡萄酒的未来价值、棒球运动员的表现、新生婴儿的健康情况,以及一对夫妇对婚姻稳定度的前景期望等问题,专家做
出的预测大错特错,令人叹为观止。
《 思考,快與慢》是預計6月15日討論的書. 不過戴老師覺得此書很無趣.讀不下去. 所以孤掌難鳴......
最近讀K. J. Wu 轉來的洪蘭教授的"負面言語讓人「未戰先敗」"
其中引用 Daniel Kahneman的一些想法
不過我認為洪教授太盡信書
.......諾貝爾經濟獎得主康納曼(D. Kahneman)在他的新書《Thinking, fast and slow 》中說,香蕉和嘔吐這兩個字本不相干,但是一旦把它們放在一起,會馬上令人感到不愉快。
大腦會自動作時間的序列,把香蕉和嘔吐連成因果,你就對香蕉產生暫時性的反感,連帶對黃色水果也不喜歡了。
這個自動化聯結所產生的作用,並不限於概念和文字,它甚至會改變你的行為。
紐約大學心理系教授巴夫請學生從五個字中選四個字出來造句,如:find(發現)、he(他)、if(如果)、yellow( 黃色)、instantly(立即)。 另一組學生看到的字則是與「老」有關,如:forgetful( 健忘的)、bald(禿頭的)、gray(灰色的)、 wrinkle(皺紋)。
做完之後,學生要到走廊另一端的實驗室去做另一個實驗。他測量學生走過走廊的時間,結果發現,那些看到跟「老」 相關字組的大學生,走的時間比看中性字組來得慢。原因在於,「 健忘」、「禿頭」、「皺紋」這些字促發了老的意念; 這個意念又促發了行為,使學生走路變慢了。
這個「促發效應」(priming effect)非常強烈。即使沒有一個學生注意到,這些字有共同的主題「老」。他們也都堅持, 老的念頭從未進入他們心中。然而,他們的行動卻變慢了, 這就是所謂的「意念動作效應」(ideomotor effect)。
更可怕的是,這個效應也可以倒過來做,動作也會強化意念。德國的研究者請學生在房間中走五分鐘,每一分鐘走三十步, 這是一般大學生步伐速度的三分之一, 然後請他們在電腦上辨認一閃而過的單字, 結果發現這些慢走的學生,對老年有關的字辨識特別快, 如forgetful、old。
假如你動作像老人,它會強化你老年的思想,這效應是雙向的。因為這暗示的作用是不自覺地發生。官員在談論國家前途時, 宜從正向著手去尋找解決方式,不可未戰先敗。........
現在我們可以查到近日心理學中的一熱門話題/爭議:Priming (psychology)
From Wikipedia, the free encyclopedia
此文批評欄中有許多異議和建議:換句話說 "the priming effects"還只是待詳細驗證的假說.Criticism
Many of the priming effects could not be replicated in further studies, casting doubt on their effectiveness or even existence.[43] Nobel Laurate and psychologist Daniel Kahneman has called on social psychologists to check the robustness of priming studies in an open letter to the community, claiming that social psychology has become a "poster child for doubts about the integrity of psychological research."[44].....
洪教授似乎過於肯定了.
-----
諾貝爾經濟學獎得主、決策大師康納曼(Daniel Kahneman)原本預計4月1日下午與桃園縣長吳志揚對談,並針對台灣公共政策及經濟環境等議題提供建言。但桃園縣政府臨時表示,康納曼疑因心臟病發,緊急送至台北榮總就醫。
▼諾貝爾獎得主康納曼原要在桃園演講,疑因心臟病發緊急送至台北榮總。(圖/取自網路)
康納曼3月31日才拜會總統馬英九討論核四問題,今日則要就桃園航空城的發展提供意見,不料會議前驚傳身體不適。《遠見雜誌》創辦人高希均下午仍舊 主持高峰論壇,他表示,康納曼早上進行研討會時站起來答覆問題,突然覺得身體不舒服,後來深怕心臟病發作,便緊急送醫,後來證實只是腸胃不適。
高希均說,康納曼目前狀況已經穩定,也託夫人打電話轉達歉意。吳志揚表示,康納曼晚間7時還要搭飛機回美國舊金山參加另一場演講,80多歲的教授還能這麼有衝勁令人佩服。
------2012.7.26
Thinking, Fast and Slow (Daniel Kahneman)
本書稱Herbert Simon 之前 加一"偉大的 "/了不起的
在Herbert Simon追思集Models of a Man中
Daniel Kahneman and Shane Frederick 合寫一篇-- Encounters with the force of Herbert A. Simon
紐約時報書評
兩個大腦在運轉,一個快一個慢
JIM HOLT 2012年07月25日
丹尼爾·卡納曼(Daniel
Kahneman)於2002年獲得諾貝爾經濟學獎。有意思的是,卡納曼是一位心理學家。具體說來,他的貢獻就在於他與另一位心理學家阿莫斯·特維斯基
(Amos Tversky(自二十世紀七十年代初開始,挑戰、瓦解了經濟學理論界長期抱持的一個概念:稱作“經濟人”(Homo
economicus)的理性優先決策者。特維斯基於1996年逝世,享年59歲。如果他還活着,他肯定會與其長期的合作者和摯友卡納曼共享諾貝爾獎。
“人類的非理性”是卡納曼的主要研究對象。他的職業生涯基本上分為三個階段。在第一階段,他和特維斯基做了一系列別出心裁的實驗,揭示了二十多個 “認知偏差”(cognitive biases)——推理中無意識的差錯歪曲了我們對世界的判斷。其中具有代表性的是“錨定效應”(anchoring effect):我們傾向於受正巧展露給我們的不相干數字的影響(例如,在一次實驗中,經驗豐富的德國法官如果擲出一對骰子後,剛好得到一個大數字,那麼 他們對商店扒手判的刑期就更長)。在第二階段,卡納曼和特維斯基證明,在不確定的情況下做決定的人,並非如傳統經濟學模型所假定的那樣行事,他們並沒有 “效用最大化”(maximize utility)。兩人隨後發展出另一種更符合人類心理的解釋決策的理論,他們稱之為“預期理論”(prospect theory,卡納曼便是因為這一成就獲得諾貝爾獎)。在其職業生涯的第三個階段——主要是在特維斯基過世以後——卡納曼轉向研究“享樂心理學” (hedonic psychology):快樂行為學及其性質和成因。他在這一領域的發現證明是令人不安的——這不僅僅是因為其中一個關鍵實驗涉及一次故意延長的結腸鏡檢 查。
《思考,快與慢》(Thinking, Fast and
Slow)的內容貫穿了以上這三個階段。這本書內容豐富,它清晰、深刻,充滿智慧的驚喜和自助價值。全書讀來妙趣橫生,在很多時候也很感人,尤其是卡納曼
講述他和特維斯基共事的時候(“我們在一起工作獲得的樂趣使我們變得格外地耐心;當你樂此不疲時,就不難做到精益求精。”)它對人類理性缺陷的洞見令人印
象深刻,《紐約時報》專欄作家大衛·布魯克斯(David
Brooks)最近就宣稱,卡納曼和特維斯基的工作“將會流芳百世”,是“我們如何看待自己的關鍵支點”。布魯克斯說,他們“就像思想界的‘路易斯與克拉
克遠征’ ”。
間接宣布了人類的非理性
現在,該說說讓我略微不安的部分。這本書的一個主題是關於過分自信。卡納曼提醒我們,我們所有人,尤其是專家,容易誇張地感覺自己是多麼了解這個世 界。當然,他自己對過分自信保持了警惕。儘管他和特維斯基(與其他研究者一道)宣稱在最近幾十年里發現了種種認知偏差、謬論和錯覺,他始終不願勇敢地宣 布,人根本就是非理性的。
抑或他做了間接的宣布?“我們大部分人在大部分時候都是健康的,我們大部分判斷和行動在大部分時候都是恰當的”,卡納曼在序言中寫道。然而,就在幾 頁之後,他又說,他和特維斯基所做的工作“挑戰”了1970年代社會學家普遍持有的觀念:“人大致是理性的。”兩位心理學家發現“在正常人的思考中存在系 統性的差錯”:差錯的出現不是源於情緒的惡劣影響,而是內置於我們逐漸演化的認知機制里。儘管卡納曼僅僅提出一些最為尋常的政策建議(例如,合同應該用更 清晰的語言表述),其他人卻發揮得更多——也許是過於自信?比如,布魯克斯認為,卡納曼和特維斯基的工作表明了“社會政策的局限”,尤其是政府為解決失業 問題、扭轉經濟局面所乾的蠢事。
這些過於籠統的結論,尚不論作者未必贊成,至少是令我皺眉的。而皺眉——你會在本書第152頁了解到——會激發我們的懷疑:懷疑卡納曼所謂的“第二 系統”。實驗表明,單是皺眉就可有效減輕過度自信;能讓我們在思考中更善於分析,更加警覺;能讓我們對那些因其輕易可得、條理井然而不假思索接受的故事產 生疑問。這就是我為什麼會皺着眉頭,持最懷疑的態度來閱讀這本非常有趣的書。
在卡納曼的模式中,第二系統是我們在思索世界時緩慢的、有意的、分析的、自覺努力的模式,第一系統與之相反,是我們快速的、自動的、直覺的、大半無 意識的模式。在一個聲音中聽到敵意,或者毫不費勁地完成“麵包和……”這個短語的是第一系統。而諸如不得不填寫納稅申報單、把車停在一個很狹小的停車位等 行為,則是第二系統在起作用(卡納曼等人發現,有一個簡單方法可以分辨一個人的第二系統在一項任務中所發揮的程度:只要盯着對方的眼睛,注意瞳孔的放大程 度)
更寬泛地說,第一系統運用聯想和隱喻快速、粗糙地勾畫出現實世界的草圖,第二系統進而達到明確的信念和理性的選擇。第一系統提出意圖,第二系統執 行。所以,第二系統似乎是老闆,對吧?在原則上是的。但第二系統不僅僅更有意圖、更加理性,同時也是懶惰的。它容易疲倦(流行的術語是“自我損耗” 【ego depletion】)。與放慢節奏、對事物進行分析相反,第二系統常常滿足於第一系統提供給它的簡單卻不可靠的關於這個世界的描述。“雖然第二系統相信 自己乃行動之所在,”卡納曼寫道:“但自動的第一系統才是本書的主角。”當你心情愉快時,第二系統似乎尤其不活躍。
這時,持懷疑態度的讀者也許會思忖,究竟要多認真地看待關於第一系統和第二系統的說法。它們真是我們頭腦中各自帶有鮮明個性的一對小小的代理人嗎?並非如此,卡納曼說道,確切地說,它們是“有用的虛構”——有用是因為它們有助於解釋人類的思維習慣。
那個叫“琳達”的銀行出納
要明白這一點,請想想“琳達問題”(the Linda problem)。卡納曼認為這是他和特維斯基一起做的“最著名和最具爭議性的”實驗。實驗的參與者會聽到一個虛構的、名叫琳達的年輕女人的故事,她單 身,坦率,非常開朗,在學生時代非常關注各種歧視和社會正義。接着,參與者會接受提問,以下哪一個更有可能:(1)琳達是一位銀行出納。(2)琳達是一位 銀行出納,活躍於女權主義運動。大多數人都選擇了(2)。換言之,提供的背景訊息表明“女權主義的銀行出納員”比“銀行出納員”更有可能。當然,這明顯違背了概率法則(每一位女權主義的銀行出納都是銀行出納;補充的細節越多,可能性就越低)。然而,甚至在斯坦福商業研究院受過大量概率訓練的學生當中,也有 百分之八十五的人無法通過琳達問題。有一位學生在得知她犯了一個低級的邏輯錯誤後,答道:“我以為你只是在詢問我的看法。”
這是怎麼回事?一個簡單的問題(敘述有多清晰連貫?)被一個更難的問題(它有多大可能?)替代了。在卡納曼看來,這就是我們在思考過程中出現許多偏 差的來源。第一系統匆匆得出一個基於“啟發”的直覺結論——這是回答艱深問題的一個簡單卻不完美的辦法——第二系統就懶惰地接受了這一啟發式的答案,絲毫 不想細查它是否合乎邏輯。
卡納曼描述了許多這類經過實驗證明的理性故障——“比率忽略”(base-rate neglect)、“有效性級聯”(availability cascade)、“有效性的錯覺”(the illusion of validity)等等。其結果就是逐漸讓讀者對人類的理性絕望。
我們真的如此無可救藥嗎?再想想琳達問題。甚至連偉大的生物進化學家斯蒂芬·傑伊·古爾德(Stephen Jay Gould)都受到它的困擾。作為一位概率專家,他知道正確的答案,但他寫道,“有一個小人兒在我頭腦里不斷地跳上跳下,對我喊道——‘可她不僅僅是一個 銀行出納;看一下描述吧。’”卡納曼使我們相信,是古爾德的第一系統一直朝他喊着錯誤的答案。可是,也許發生着更為微妙的事情。我們的日常對話都發生在一 個對期望未加說明的豐富背景下——語言學家稱之為“言下之意”(implicatures)。這些言下之意可以滲透到心理實驗中去。鑒於我們都期望讓對話 更加簡潔,實驗的參與者將“琳達是一位銀行職”當作是在暗示“此外,她並不是一個女權主義者”,也是相當合理的。如果是這樣,他們的答案就並非那麼謬誤。
這似乎是一個次要的問題。但它卻適用於卡納曼和特維斯基,還有其他研究者所聲稱的在正規的實驗中發現的一些偏差。在更加自然的情景中——當我們在偵 查騙子而不是解決邏輯謎題,在推斷事物而不是象徵物,在評估原始數據而不是百分比的時候——人們就不太可能出現同樣的差錯。至少,後來的許多實驗都間接地 表明了這一點。也許我們根本就不是那麼不理性。
當然,一些認知偏差甚至公然出現在最為自然的情景中。比如卡納曼所說的“規劃謬誤”(planning fallacy):我們傾向於高估利潤和低估成本,因而愚蠢地施行一些存在很大風險的方案。例如,在2002年,美國人改建廚房,預期這項工作平均花費 18,658美元,但他們最後卻花費了38,769美元。
"規劃謬誤“只是一種普遍存在的樂觀偏差的一個表現”,卡納曼寫道,這“很可能是最重大的認知偏差”。在某種意義上,一種傾向於樂觀主義的偏差顯然是 糟糕的,因為它帶來錯誤的信念——比如:是我們在掌控運氣而不是運氣在玩弄我們。但是,如果沒有這一“掌控的錯覺”,我們在早上甚至都沒辦法起床吧?比起 與之相對應的更立足於現實的人,樂觀主義者更具心理彈性,具備更強大的免疫系統,平均壽命更長。此外,正如卡納曼所指出的,過分的樂觀主義使個人和組織都 免受另一種偏差的麻痹效應,這種偏差就是“損失規避”(loss aversion):我們對損失的畏懼更甚於對獲利的重視。當約翰·梅納爾德·凱恩斯(John Maynard Keynes)談到驅策資本主義的“動物精神”(animal spirits)時,他頭腦中就存在着過分的樂觀主義。
即便我們能夠擺脫這本書所指出的那些偏差和錯覺——卡納曼以他自己在克服這些偏差和錯覺方面殊少進步為例,懷疑我們也不能克服它們——我們也根本不 清楚這是否能讓我們的生活變得更好。這引發了一個根本問題:理性的意義何在?說到底,我們全都是達爾文學說里的倖存者。我們日常的推理能力為了有效地適應 一個複雜的動態環境,已經隨之進化了。因此,這些推理能力大概也會適應這一環境,即便它們在心理學家那些多少存在人為因素的實驗中出錯。如果理性的模範不 是對人類在日常生活中的實際推理的一種理想化,那它們又是從何而來?作為一個物種,我們不能讓自己的判斷存在普遍的偏差,就像我們不能在使用語言時普遍地 不顧語法——抑或在對像卡納曼和特維斯基所做的研究進行批評時也是如此。
幸福是什麼?
卡納曼從未從哲學上抓住理性的特徵。然而,他為能夠作為其目標的幸福提供了一個迷人的描述。幸福是什麼意思?當卡納曼在1990年代中期首次提出這 個問題時,大部分對幸福的研究還依賴於詢問人們在大體上對他們的生活感到多麼滿意。但這種回顧性的評估依賴於記憶,眾所周知,記憶是不可靠的。如果與此相 反,一個人對快樂或者痛苦的實際體驗能夠隨時隨地取樣,然後隨着時間推移加以總結,那會怎樣?卡納曼將之稱為“體驗的”幸福,與研究者依賴的“記憶的”幸 福相對立。他發現這兩種對幸福的衡量方式存在驚人的差異。使“體驗的自我”(experiencing self)感到幸福的東西並不是使“記憶的自我”(remembering self)感到幸福的東西。尤其是,記憶的自我並不在乎持續時間——不在乎一段愉快或者不愉快的經歷持續多久。它會通過體驗過程中痛苦或者快樂的峰值水 平,通過體驗的結果來回顧性地衡量一段體驗。
記憶的幸福的兩個缺陷——“對持續時間的忽略”(duration neglect)和“峰終定律”(peak-end rule)——在卡納曼的一個更令人難受的實驗中得到了驚人的展現。兩組病人要接受痛苦的結腸鏡檢查。A組病人依照的是正常的程序。B組病人也一樣,只不 過——他們沒被告知——在檢查結束後,額外加上了幾分鐘的輕度不適。哪一組更痛苦呢?嗯,B組承受了A組的全部痛苦,然後還有額外的一些痛苦。但由於B組 的結腸鏡檢查延長意味着結束時的痛苦要小於A組,這一組病人在回顧的時候就不那麼在意(在更早的一篇研究論文中——雖然不在這本書里——卡納曼提出,在這 個實驗中,如果B組受到的這陣額外的不適能夠增強他們回來參加後續實驗的意願,它就是合乎倫理的!)。
在結腸鏡檢查中是如此,在生活中也是如此。在發號施令的是記憶的自我,而不是體驗的自我。例如,卡納曼引述研究表明,一個大學生決定是否重複一次春 季假期,取決於前一個假期的峰終定律(peak-end rule),而不是取決於那一個一個瞬間多麼有趣(或者多麼悲慘)。記憶的自我對無聲的體驗的自我施加了一種“暴虐”。“好像很奇怪,”卡納曼寫道,“我 是我記憶的自我,而那個過着我的生活的體驗的自我,對我而言就像一個陌生人。”
卡納曼的結論聽起來很激進,也許還不夠激進。也許根本就沒有體驗的自我。例如,以色列魏慈曼研究所(Weizmann Institute)的拉斐爾·馬拉克(Rafael Malach)和同事進行的大腦掃描實驗表明,當實驗對象專註於一項體驗,比如觀看電影《黃金三鏢客》(The Good, the Bad, and the Ugly),大腦中跟自我意識聯繫起來的部分不僅僅是安靜了,實際上是被大腦的其他部分關閉(“抑制”)了。自我似乎消失了。那麼,到底是誰在享受電影 呢?為什麼這種無我的快樂會進入記憶的自我的決策演算中呢?
顯然,在享樂心理學中還有許多研究要做。但卡納曼的觀念革新已經為他在書中講到的許多經驗成果打下了基礎:較之美國母親,法國母親與子女待在一起的 時間更少,但她們卻更享受;窮人更受頭痛的侵襲;獨居的女人似乎與那些有配偶的女人享受着同等的幸福;在這個國家的高生活成本地區,一個收入大約 75,000美元的家庭足夠將幸福最大化。那些有志於降低社會不幸指數的政策制定者,將會在這裡發現許多值得深思的東西。
在讀到《思考,快與慢》結尾的時候,我充滿狐疑的皺眉早已舒展開,臉上掛着智性滿足的微笑。根據峰終定律來評價這本書,我會過分自信地催促每個人都 去買來讀。但對於那些只關心卡納曼如何售賣馬爾科姆·格拉德維爾問題的人來說:如果你已經在一個可預知的、快速反饋的環境中接受了10,000小時的訓練 ——國際象棋、消防、麻醉學——那麼,就不用看了。至於其他人,則好好想想吧。
“人類的非理性”是卡納曼的主要研究對象。他的職業生涯基本上分為三個階段。在第一階段,他和特維斯基做了一系列別出心裁的實驗,揭示了二十多個 “認知偏差”(cognitive biases)——推理中無意識的差錯歪曲了我們對世界的判斷。其中具有代表性的是“錨定效應”(anchoring effect):我們傾向於受正巧展露給我們的不相干數字的影響(例如,在一次實驗中,經驗豐富的德國法官如果擲出一對骰子後,剛好得到一個大數字,那麼 他們對商店扒手判的刑期就更長)。在第二階段,卡納曼和特維斯基證明,在不確定的情況下做決定的人,並非如傳統經濟學模型所假定的那樣行事,他們並沒有 “效用最大化”(maximize utility)。兩人隨後發展出另一種更符合人類心理的解釋決策的理論,他們稱之為“預期理論”(prospect theory,卡納曼便是因為這一成就獲得諾貝爾獎)。在其職業生涯的第三個階段——主要是在特維斯基過世以後——卡納曼轉向研究“享樂心理學” (hedonic psychology):快樂行為學及其性質和成因。他在這一領域的發現證明是令人不安的——這不僅僅是因為其中一個關鍵實驗涉及一次故意延長的結腸鏡檢 查。
相關文章
間接宣布了人類的非理性
現在,該說說讓我略微不安的部分。這本書的一個主題是關於過分自信。卡納曼提醒我們,我們所有人,尤其是專家,容易誇張地感覺自己是多麼了解這個世 界。當然,他自己對過分自信保持了警惕。儘管他和特維斯基(與其他研究者一道)宣稱在最近幾十年里發現了種種認知偏差、謬論和錯覺,他始終不願勇敢地宣 布,人根本就是非理性的。
抑或他做了間接的宣布?“我們大部分人在大部分時候都是健康的,我們大部分判斷和行動在大部分時候都是恰當的”,卡納曼在序言中寫道。然而,就在幾 頁之後,他又說,他和特維斯基所做的工作“挑戰”了1970年代社會學家普遍持有的觀念:“人大致是理性的。”兩位心理學家發現“在正常人的思考中存在系 統性的差錯”:差錯的出現不是源於情緒的惡劣影響,而是內置於我們逐漸演化的認知機制里。儘管卡納曼僅僅提出一些最為尋常的政策建議(例如,合同應該用更 清晰的語言表述),其他人卻發揮得更多——也許是過於自信?比如,布魯克斯認為,卡納曼和特維斯基的工作表明了“社會政策的局限”,尤其是政府為解決失業 問題、扭轉經濟局面所乾的蠢事。
這些過於籠統的結論,尚不論作者未必贊成,至少是令我皺眉的。而皺眉——你會在本書第152頁了解到——會激發我們的懷疑:懷疑卡納曼所謂的“第二 系統”。實驗表明,單是皺眉就可有效減輕過度自信;能讓我們在思考中更善於分析,更加警覺;能讓我們對那些因其輕易可得、條理井然而不假思索接受的故事產 生疑問。這就是我為什麼會皺着眉頭,持最懷疑的態度來閱讀這本非常有趣的書。
在卡納曼的模式中,第二系統是我們在思索世界時緩慢的、有意的、分析的、自覺努力的模式,第一系統與之相反,是我們快速的、自動的、直覺的、大半無 意識的模式。在一個聲音中聽到敵意,或者毫不費勁地完成“麵包和……”這個短語的是第一系統。而諸如不得不填寫納稅申報單、把車停在一個很狹小的停車位等 行為,則是第二系統在起作用(卡納曼等人發現,有一個簡單方法可以分辨一個人的第二系統在一項任務中所發揮的程度:只要盯着對方的眼睛,注意瞳孔的放大程 度)
更寬泛地說,第一系統運用聯想和隱喻快速、粗糙地勾畫出現實世界的草圖,第二系統進而達到明確的信念和理性的選擇。第一系統提出意圖,第二系統執 行。所以,第二系統似乎是老闆,對吧?在原則上是的。但第二系統不僅僅更有意圖、更加理性,同時也是懶惰的。它容易疲倦(流行的術語是“自我損耗” 【ego depletion】)。與放慢節奏、對事物進行分析相反,第二系統常常滿足於第一系統提供給它的簡單卻不可靠的關於這個世界的描述。“雖然第二系統相信 自己乃行動之所在,”卡納曼寫道:“但自動的第一系統才是本書的主角。”當你心情愉快時,第二系統似乎尤其不活躍。
這時,持懷疑態度的讀者也許會思忖,究竟要多認真地看待關於第一系統和第二系統的說法。它們真是我們頭腦中各自帶有鮮明個性的一對小小的代理人嗎?並非如此,卡納曼說道,確切地說,它們是“有用的虛構”——有用是因為它們有助於解釋人類的思維習慣。
那個叫“琳達”的銀行出納
要明白這一點,請想想“琳達問題”(the Linda problem)。卡納曼認為這是他和特維斯基一起做的“最著名和最具爭議性的”實驗。實驗的參與者會聽到一個虛構的、名叫琳達的年輕女人的故事,她單 身,坦率,非常開朗,在學生時代非常關注各種歧視和社會正義。接着,參與者會接受提問,以下哪一個更有可能:(1)琳達是一位銀行出納。(2)琳達是一位 銀行出納,活躍於女權主義運動。大多數人都選擇了(2)。換言之,提供的背景訊息表明“女權主義的銀行出納員”比“銀行出納員”更有可能。當然,這明顯違背了概率法則(每一位女權主義的銀行出納都是銀行出納;補充的細節越多,可能性就越低)。然而,甚至在斯坦福商業研究院受過大量概率訓練的學生當中,也有 百分之八十五的人無法通過琳達問題。有一位學生在得知她犯了一個低級的邏輯錯誤後,答道:“我以為你只是在詢問我的看法。”
這是怎麼回事?一個簡單的問題(敘述有多清晰連貫?)被一個更難的問題(它有多大可能?)替代了。在卡納曼看來,這就是我們在思考過程中出現許多偏 差的來源。第一系統匆匆得出一個基於“啟發”的直覺結論——這是回答艱深問題的一個簡單卻不完美的辦法——第二系統就懶惰地接受了這一啟發式的答案,絲毫 不想細查它是否合乎邏輯。
卡納曼描述了許多這類經過實驗證明的理性故障——“比率忽略”(base-rate neglect)、“有效性級聯”(availability cascade)、“有效性的錯覺”(the illusion of validity)等等。其結果就是逐漸讓讀者對人類的理性絕望。
我們真的如此無可救藥嗎?再想想琳達問題。甚至連偉大的生物進化學家斯蒂芬·傑伊·古爾德(Stephen Jay Gould)都受到它的困擾。作為一位概率專家,他知道正確的答案,但他寫道,“有一個小人兒在我頭腦里不斷地跳上跳下,對我喊道——‘可她不僅僅是一個 銀行出納;看一下描述吧。’”卡納曼使我們相信,是古爾德的第一系統一直朝他喊着錯誤的答案。可是,也許發生着更為微妙的事情。我們的日常對話都發生在一 個對期望未加說明的豐富背景下——語言學家稱之為“言下之意”(implicatures)。這些言下之意可以滲透到心理實驗中去。鑒於我們都期望讓對話 更加簡潔,實驗的參與者將“琳達是一位銀行職”當作是在暗示“此外,她並不是一個女權主義者”,也是相當合理的。如果是這樣,他們的答案就並非那麼謬誤。
這似乎是一個次要的問題。但它卻適用於卡納曼和特維斯基,還有其他研究者所聲稱的在正規的實驗中發現的一些偏差。在更加自然的情景中——當我們在偵 查騙子而不是解決邏輯謎題,在推斷事物而不是象徵物,在評估原始數據而不是百分比的時候——人們就不太可能出現同樣的差錯。至少,後來的許多實驗都間接地 表明了這一點。也許我們根本就不是那麼不理性。
當然,一些認知偏差甚至公然出現在最為自然的情景中。比如卡納曼所說的“規劃謬誤”(planning fallacy):我們傾向於高估利潤和低估成本,因而愚蠢地施行一些存在很大風險的方案。例如,在2002年,美國人改建廚房,預期這項工作平均花費 18,658美元,但他們最後卻花費了38,769美元。
"規劃謬誤“只是一種普遍存在的樂觀偏差的一個表現”,卡納曼寫道,這“很可能是最重大的認知偏差”。在某種意義上,一種傾向於樂觀主義的偏差顯然是 糟糕的,因為它帶來錯誤的信念——比如:是我們在掌控運氣而不是運氣在玩弄我們。但是,如果沒有這一“掌控的錯覺”,我們在早上甚至都沒辦法起床吧?比起 與之相對應的更立足於現實的人,樂觀主義者更具心理彈性,具備更強大的免疫系統,平均壽命更長。此外,正如卡納曼所指出的,過分的樂觀主義使個人和組織都 免受另一種偏差的麻痹效應,這種偏差就是“損失規避”(loss aversion):我們對損失的畏懼更甚於對獲利的重視。當約翰·梅納爾德·凱恩斯(John Maynard Keynes)談到驅策資本主義的“動物精神”(animal spirits)時,他頭腦中就存在着過分的樂觀主義。
即便我們能夠擺脫這本書所指出的那些偏差和錯覺——卡納曼以他自己在克服這些偏差和錯覺方面殊少進步為例,懷疑我們也不能克服它們——我們也根本不 清楚這是否能讓我們的生活變得更好。這引發了一個根本問題:理性的意義何在?說到底,我們全都是達爾文學說里的倖存者。我們日常的推理能力為了有效地適應 一個複雜的動態環境,已經隨之進化了。因此,這些推理能力大概也會適應這一環境,即便它們在心理學家那些多少存在人為因素的實驗中出錯。如果理性的模範不 是對人類在日常生活中的實際推理的一種理想化,那它們又是從何而來?作為一個物種,我們不能讓自己的判斷存在普遍的偏差,就像我們不能在使用語言時普遍地 不顧語法——抑或在對像卡納曼和特維斯基所做的研究進行批評時也是如此。
幸福是什麼?
卡納曼從未從哲學上抓住理性的特徵。然而,他為能夠作為其目標的幸福提供了一個迷人的描述。幸福是什麼意思?當卡納曼在1990年代中期首次提出這 個問題時,大部分對幸福的研究還依賴於詢問人們在大體上對他們的生活感到多麼滿意。但這種回顧性的評估依賴於記憶,眾所周知,記憶是不可靠的。如果與此相 反,一個人對快樂或者痛苦的實際體驗能夠隨時隨地取樣,然後隨着時間推移加以總結,那會怎樣?卡納曼將之稱為“體驗的”幸福,與研究者依賴的“記憶的”幸 福相對立。他發現這兩種對幸福的衡量方式存在驚人的差異。使“體驗的自我”(experiencing self)感到幸福的東西並不是使“記憶的自我”(remembering self)感到幸福的東西。尤其是,記憶的自我並不在乎持續時間——不在乎一段愉快或者不愉快的經歷持續多久。它會通過體驗過程中痛苦或者快樂的峰值水 平,通過體驗的結果來回顧性地衡量一段體驗。
記憶的幸福的兩個缺陷——“對持續時間的忽略”(duration neglect)和“峰終定律”(peak-end rule)——在卡納曼的一個更令人難受的實驗中得到了驚人的展現。兩組病人要接受痛苦的結腸鏡檢查。A組病人依照的是正常的程序。B組病人也一樣,只不 過——他們沒被告知——在檢查結束後,額外加上了幾分鐘的輕度不適。哪一組更痛苦呢?嗯,B組承受了A組的全部痛苦,然後還有額外的一些痛苦。但由於B組 的結腸鏡檢查延長意味着結束時的痛苦要小於A組,這一組病人在回顧的時候就不那麼在意(在更早的一篇研究論文中——雖然不在這本書里——卡納曼提出,在這 個實驗中,如果B組受到的這陣額外的不適能夠增強他們回來參加後續實驗的意願,它就是合乎倫理的!)。
在結腸鏡檢查中是如此,在生活中也是如此。在發號施令的是記憶的自我,而不是體驗的自我。例如,卡納曼引述研究表明,一個大學生決定是否重複一次春 季假期,取決於前一個假期的峰終定律(peak-end rule),而不是取決於那一個一個瞬間多麼有趣(或者多麼悲慘)。記憶的自我對無聲的體驗的自我施加了一種“暴虐”。“好像很奇怪,”卡納曼寫道,“我 是我記憶的自我,而那個過着我的生活的體驗的自我,對我而言就像一個陌生人。”
卡納曼的結論聽起來很激進,也許還不夠激進。也許根本就沒有體驗的自我。例如,以色列魏慈曼研究所(Weizmann Institute)的拉斐爾·馬拉克(Rafael Malach)和同事進行的大腦掃描實驗表明,當實驗對象專註於一項體驗,比如觀看電影《黃金三鏢客》(The Good, the Bad, and the Ugly),大腦中跟自我意識聯繫起來的部分不僅僅是安靜了,實際上是被大腦的其他部分關閉(“抑制”)了。自我似乎消失了。那麼,到底是誰在享受電影 呢?為什麼這種無我的快樂會進入記憶的自我的決策演算中呢?
顯然,在享樂心理學中還有許多研究要做。但卡納曼的觀念革新已經為他在書中講到的許多經驗成果打下了基礎:較之美國母親,法國母親與子女待在一起的 時間更少,但她們卻更享受;窮人更受頭痛的侵襲;獨居的女人似乎與那些有配偶的女人享受着同等的幸福;在這個國家的高生活成本地區,一個收入大約 75,000美元的家庭足夠將幸福最大化。那些有志於降低社會不幸指數的政策制定者,將會在這裡發現許多值得深思的東西。
在讀到《思考,快與慢》結尾的時候,我充滿狐疑的皺眉早已舒展開,臉上掛着智性滿足的微笑。根據峰終定律來評價這本書,我會過分自信地催促每個人都 去買來讀。但對於那些只關心卡納曼如何售賣馬爾科姆·格拉德維爾問題的人來說:如果你已經在一個可預知的、快速反饋的環境中接受了10,000小時的訓練 ——國際象棋、消防、麻醉學——那麼,就不用看了。至於其他人,則好好想想吧。
吉姆·霍爾特(Jim Holt )的新書叫《世界為什麼存在?》(“Why Does the World Exist?”)。
本文最初發表於2011年11月25日。
翻譯:流暢
2013年5月27日 星期一
Preface to the Chinese Translation of The Sciences of the Artificial 『人工科學通識』中文版序
這篇Simon的序是從2000年的網站所搶救的.
www.deming.com.tw
每周至少五天更新:2000/07/17(updated July 17,
2000Thanks(鳴謝)
感謝司馬賀(H. A. Simon)教授贈其重要論文" Economics As A Historical
Science",本刊將陸續簡介。並賜 『人工科學通識』中文版序
Science",本刊將陸續簡介。並賜 『人工科學通識』中文版序
Preface to the Chinese Translation;It is a great pleasure to me to see The Sciences of the Artificial made available in the Chinese language.In the kind of world in which we live today, we can no longer afford the luxury of limiting new knowledge to the particular language community in which it happens to originate. We understand today that knowledge, not material things, is the principal basis for human productivity and for the problem solving skills that we need to continue to live, with each other and with Nature, on our Earth.
Full exchange of knowledge between the Chinese and English language communities is especially important, because these are the most widely used languages world, and books that are published in both tongues will thereby become available to most of the world's people.
I wish to thank Mr. Hanching Chung warmly for his vigorous efforts both to translate The
Sciences of the Artificial and to arrange for its publication I hope that readers of the Chinese language edition will be encouraged to join in the exciting adventure of exploring the nature of the systems that play such a large role in our modern world
Herbert A. Simon
Pittsburgh, Pennsylvania, U.S.A
June 24, 2000
翻譯是深層游戲
近月來投入翻譯工作,相當的辛苦,不過甘苦參半,有時也很快活
我出版的翻譯本,一定比原文豐富:不只有原作者序、譯注、譯後報
real Simon Pure.=the real or genuine person or
article可見我的司馬賀叢書是「真貨」。這位大科學家最擅
計畫學、複雜系統、決策 問題解決學、……)」一以貫之,所以我將這本書名取為《人工科
請看目錄第一章
了解自然和人工世界
第二章
經濟的理性
第三章思考的心理學:在自然中深埋人造物
第四章記起與學習記憶作為思想的環境
第五章設計的科學
第六章社會計劃:設計演化中的人造物
第七章關於複雜性的各種觀點複雜性結構:各種層級系統
第六章社會計劃:設計演化中的人造物
第七章關於複雜性的各種觀點複雜性結構:各種層級系統
2013年5月4日 星期六
關於"The Tacit Dimension"的評說
最近台灣有書 名常識
是想仿近300年前美國的暢銷書?
其實現在科學/心理學等大興 一常識 等都可以深入分析: 為什麼知識或迷信等變成常識
---
我曾請教Herbert Simon 關於 tacit knowledge 方面的想法
他是科學家 認為Michael Polanyi 的招牌說法是沒什麼"神秘"可言 現在人們知道得更多
Polanyi's concept of tacit knowledge was first articulated in Personal Knowledge.
... published as "The Tacit Dimension" (1966) he seeks to distinguish between ...
".....此外,「藝師藝友」,因為都是藝文界的知名人物,實即大家的藝師藝友,有的大家已經忘卻,今日重睹故人,會憶起他們對今日台灣的貢獻, 會油然萌生親切之感。「逆旅形色」在旅遊發達的今天,你會覺得「到此一遊」的雪泥鴻爪,也可作藝術創作的處理,別死命的盯著一個風物,把握瞬間稍縱即逝的 機會,憑感覺快速的喀擦,那意外的頃刻,便適時留下了它飛逝中的停格。「人間偶遇」,也是值得參考的作品。不然一生中多少側身而過的偶然,若感覺是新鮮 的,所有的偶然,說不定都是永恆。
在巴黎,朱德群夫人景昭女士,問我作畫時,都有沒有計劃?我說「沒有!」面對畫面的空白一、二秒鐘的蓄勢,便開始畫到哪是哪。一切都是遇然的機緣,畫完了,有時意猶未足,說不定,隨意的在某個角落墮入一點。她說「德群也是這樣」沒有預設的計劃。
想不到攝影家莊靈,也全憑那瞬間感覺的靈視,使擦身而過、陌生的異鄉偶然,變成了他的必然。「心照自然」也是在這樣的心情下,即興般的喀擦,「非由造作,畫法自然」的成果,都是「泥上偶然留指爪」和回顧哪復計東西耶。"楚戈
這些類似的文學藝術家哲學家等人的"直覺"或 TACIT DIMENTION等的看法
Simon認為那極可能沒有深入研究的假設
我有封信跟他略談 他的論點之摘要都寫在 "管理行為"
是想仿近300年前美國的暢銷書?
其實現在科學/心理學等大興 一常識 等都可以深入分析: 為什麼知識或迷信等變成常識
---
我曾請教Herbert Simon 關於 tacit knowledge 方面的想法
他是科學家 認為Michael Polanyi 的招牌說法是沒什麼"神秘"可言 現在人們知道得更多
Polanyi's concept of tacit knowledge was first articulated in Personal Knowledge.
... published as "The Tacit Dimension" (1966) he seeks to distinguish between ...
".....此外,「藝師藝友」,因為都是藝文界的知名人物,實即大家的藝師藝友,有的大家已經忘卻,今日重睹故人,會憶起他們對今日台灣的貢獻, 會油然萌生親切之感。「逆旅形色」在旅遊發達的今天,你會覺得「到此一遊」的雪泥鴻爪,也可作藝術創作的處理,別死命的盯著一個風物,把握瞬間稍縱即逝的 機會,憑感覺快速的喀擦,那意外的頃刻,便適時留下了它飛逝中的停格。「人間偶遇」,也是值得參考的作品。不然一生中多少側身而過的偶然,若感覺是新鮮 的,所有的偶然,說不定都是永恆。
在巴黎,朱德群夫人景昭女士,問我作畫時,都有沒有計劃?我說「沒有!」面對畫面的空白一、二秒鐘的蓄勢,便開始畫到哪是哪。一切都是遇然的機緣,畫完了,有時意猶未足,說不定,隨意的在某個角落墮入一點。她說「德群也是這樣」沒有預設的計劃。
想不到攝影家莊靈,也全憑那瞬間感覺的靈視,使擦身而過、陌生的異鄉偶然,變成了他的必然。「心照自然」也是在這樣的心情下,即興般的喀擦,「非由造作,畫法自然」的成果,都是「泥上偶然留指爪」和回顧哪復計東西耶。"楚戈
這些類似的文學藝術家哲學家等人的"直覺"或 TACIT DIMENTION等的看法
Simon認為那極可能沒有深入研究的假設
我有封信跟他略談 他的論點之摘要都寫在 "管理行為"
Hanching Chung 這本書有一故事 某中研院院士的指導教授是海耶克. Hayek建議他寫這. 他搞不懂.所以放棄. 他回台灣一直強調此書(論文集)不可翻譯.....我跟Herbert Simon談過tacit knowledge 他說現在心理學對此已有很多科學知識......
2013年5月1日 星期三
Deep Learning /人工智慧新境What Is Artificial Intelligence? By RICHARD POWERS Published: February 5, 2011
Deep Learning
With massive amounts of computational power, machines can now recognize objects and translate speech in real time. Artificial intelligence is finally getting smart.
AI這 行業採用的術語似乎很誇張 譬如說所謂的 Deep Learning
«
»
Deep Learning
With massive amounts of computational power, machines can now recognize objects and translate speech in real time. Artificial intelligence is finally getting smart.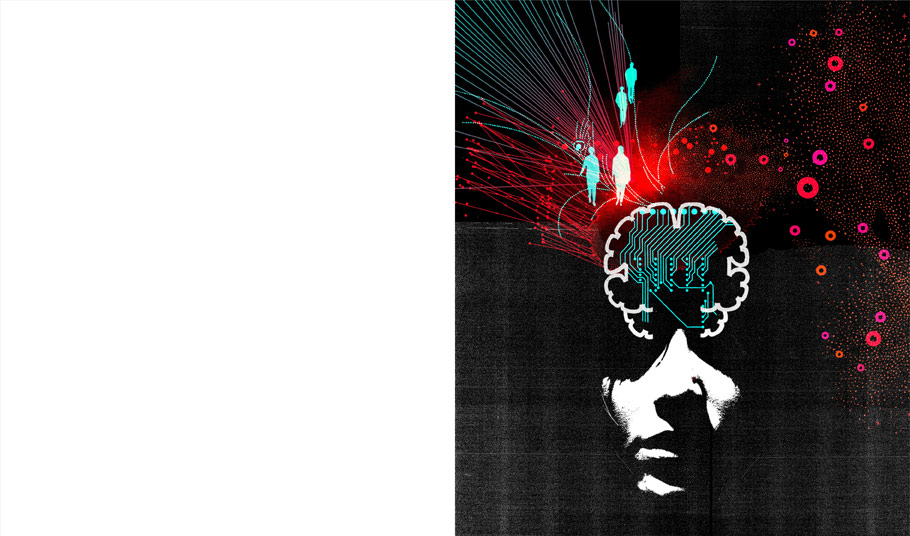
When Ray Kurzweil met with Google CEO Larry Page last
July, he wasn’t looking for a job. A respected inventor who’s become a
machine-intelligence futurist, Kurzweil wanted to discuss his upcoming
book How to Create a Mind. He told Page, who had read an early
draft, that he wanted to start a company to develop his ideas about how
to build a truly intelligent computer: one that could understand
language and then make inferences and decisions on its own.
Kurzweil was attracted not just by Google’s computing resources but also by the startling progress the company has made in a branch of AI called deep learning. Deep-learning software attempts to mimic the activity in layers of neurons in the neocortex, the wrinkly 80 percent of the brain where thinking occurs. The software learns, in a very real sense, to recognize patterns in digital representations of sounds, images, and other data.
The basic idea—that software can simulate the neocortex’s large array of neurons in an artificial “neural network”—is decades old, and it has led to as many disappointments as breakthroughs. But because of improvements in mathematical formulas and increasingly powerful computers, computer scientists can now model many more layers of virtual neurons than ever before.
With this greater depth, they are producing remarkable advances in speech and image recognition. Last June, a Google deep-learning system that had been shown 10 million images from YouTube videos proved almost twice as good as any previous image recognition effort at identifying objects such as cats. Google also used the technology to cut the error rate on speech recognition in its latest Android mobile software. In October, Microsoft chief research officer Rick Rashid wowed attendees at a lecture in China with a demonstration of speech software that transcribed his spoken words into English text with an error rate of 7 percent, translated them into Chinese-language text, and then simulated his own voice uttering them in Mandarin. That same month, a team of three graduate students and two professors won a contest held by Merck to identify molecules that could lead to new drugs. The group used deep learning to zero in on the molecules most likely to bind to their targets.
Google in particular has become a magnet for deep learning and related AI talent. In March the company bought a startup cofounded by Geoffrey Hinton, a University of Toronto computer science professor who was part of the team that won the Merck contest. Hinton, who will split his time between the university and Google, says he plans to “take ideas out of this field and apply them to real problems” such as image recognition, search, and natural-language understanding, he says.
All this has normally cautious AI researchers hopeful that intelligent machines may finally escape the pages of science fiction. Indeed, machine intelligence is starting to transform everything from communications and computing to medicine, manufacturing, and transportation. The possibilities are apparent in IBM’s Jeopardy!-winning Watson computer, which uses some deep-learning techniques and is now being trained to help doctors make better decisions. Microsoft has deployed deep learning in its Windows Phone and Bing voice search.
Extending deep learning into applications beyond speech and image recognition will require more conceptual and software breakthroughs, not to mention many more advances in processing power. And we probably won’t see machines we all agree can think for themselves for years, perhaps decades—if ever. But for now, says Peter Lee, head of Microsoft Research USA, “deep learning has reignited some of the grand challenges in artificial intelligence.”
Building a Brain
There have been many competing approaches to those challenges. One has been to feed computers with information and rules about the world, which required programmers to laboriously write software that is familiar with the attributes of, say, an edge or a sound. That took lots of time and still left the systems unable to deal with ambiguous data; they were limited to narrow, controlled applications such as phone menu systems that ask you to make queries by saying specific words.
Neural networks, developed in the 1950s not long after the dawn of AI research, looked promising because they attempted to simulate the way the brain worked, though in greatly simplified form. A program maps out a set of virtual neurons and then assigns random numerical values, or “weights,” to connections between them. These weights determine how each simulated neuron responds—with a mathematical output between 0 and 1—to a digitized feature such as an edge or a shade of blue in an image, or a particular energy level at one frequency in a phoneme, the individual unit of sound in spoken syllables.
Some of today’s artificial neural networks can train themselves to recognize complex patterns.Programmers would train a neural network to detect an object or phoneme by blitzing the network with digitized versions of images containing those objects or sound waves containing those phonemes. If the network didn’t accurately recognize a particular pattern, an algorithm would adjust the weights. The eventual goal of this training was to get the network to consistently recognize the patterns in speech or sets of images that we humans know as, say, the phoneme “d” or the image of a dog. This is much the same way a child learns what a dog is by noticing the details of head shape, behavior, and the like in furry, barking animals that other people call dogs.
But early neural networks could simulate only a very limited number of neurons at once, so they could not recognize patterns of great complexity. They languished through the 1970s.

In the mid-1980s, Hinton and others helped spark a revival of interest in neural networks with so-called “deep” models that made better use of many layers of software neurons. But the technique still required heavy human involvement: programmers had to label data before feeding it to the network. And complex speech or image recognition required more computer power than was then available.
Finally, however, in the last decade Hinton and other researchers made some fundamental conceptual breakthroughs. In 2006, Hinton developed a more efficient way to teach individual layers of neurons. The first layer learns primitive features, like an edge in an image or the tiniest unit of speech sound. It does this by finding combinations of digitized pixels or sound waves that occur more often than they should by chance. Once that layer accurately recognizes those features, they’re fed to the next layer, which trains itself to recognize more complex features, like a corner or a combination of speech sounds. The process is repeated in successive layers until the system can reliably recognize phonemes or objects.
Like cats. Last June, Google demonstrated one of the largest neural networks yet, with more than a billion connections. A team led by Stanford computer science professor Andrew Ng and Google Fellow Jeff Dean showed the system images from 10 million randomly selected YouTube videos. One simulated neuron in the software model fixated on images of cats. Others focused on human faces, yellow flowers, and other objects. And thanks to the power of deep learning, the system identified these discrete objects even though no humans had ever defined or labeled them.
What stunned some AI experts, though, was the magnitude of improvement in image recognition. The system correctly categorized objects and themes in the YouTube images 16 percent of the time. That might not sound impressive, but it was 70 percent better than previous methods. And, Dean notes, there were 22,000 categories to choose from; correctly slotting objects into some of them required, for example, distinguishing between two similar varieties of skate fish. That would have been challenging even for most humans. When the system was asked to sort the images into 1,000 more general categories, the accuracy rate jumped above 50 percent.
Big Data
Training the many layers of virtual neurons in the experiment took 16,000 computer processors—the kind of computing infrastructure that Google has developed for its search engine and other services. At least 80 percent of the recent advances in AI can be attributed to the availability of more computer power, reckons Dileep George, cofounder of the machine-learning startup Vicarious.
There’s more to it than the sheer size of Google’s data centers, though. Deep learning has also benefited from the company’s method of splitting computing tasks among many machines so they can be done much more quickly. That’s a technology Dean helped develop earlier in his 14-year career at Google. It vastly speeds up the training of deep-learning neural networks as well, enabling Google to run larger networks and feed a lot more data to them.
Already, deep learning has improved voice search on smartphones. Until last year, Google’s Android software used a method that misunderstood many words. But in preparation for a new release of Android last July, Dean and his team helped replace part of the speech system with one based on deep learning. Because the multiple layers of neurons allow for more precise training on the many variants of a sound, the system can recognize scraps of sound more reliably, especially in noisy environments such as subway platforms. Since it’s likelier to understand what was actually uttered, the result it returns is likelier to be accurate as well. Almost overnight, the number of errors fell by up to 25 percent—results so good that many reviewers now deem Android’s voice search smarter than Apple’s more famous Siri voice assistant.
For all the advances, not everyone thinks deep learning can move artificial intelligence toward something rivaling human intelligence. Some critics say deep learning and AI in general ignore too much of the brain’s biology in favor of brute-force computing.
One such critic is Jeff Hawkins, founder of Palm Computing, whose latest venture, Numenta, is developing a machine-learning system that is biologically inspired but does not use deep learning. Numenta’s system can help predict energy consumption patterns and the likelihood that a machine such as a windmill is about to fail. Hawkins, author of On Intelligence, a 2004 book on how the brain works and how it might provide a guide to building intelligent machines, says deep learning fails to account for the concept of time. Brains process streams of sensory data, he says, and human learning depends on our ability to recall sequences of patterns: when you watch a video of a cat doing something funny, it’s the motion that matters, not a series of still images like those Google used in its experiment. “Google’s attitude is: lots of data makes up for everything,” Hawkins says.
But if it doesn’t make up for everything, the computing resources a company like Google throws at these problems can’t be dismissed. They’re crucial, say deep-learning advocates, because the brain itself is still so much more complex than any of today’s neural networks. “You need lots of computational resources to make the ideas work at all,” says Hinton.
What’s Next
Although Google is less than forthcoming about future applications, the prospects are intriguing. Clearly, better image search would help YouTube, for instance. And Dean says deep-learning models can use phoneme data from English to more quickly train systems to recognize the spoken sounds in other languages. It’s also likely that more sophisticated image recognition could make Google’s self-driving cars much better. Then there’s search and the ads that underwrite it. Both could see vast improvements from any technology that’s better and faster at recognizing what people are really looking for—maybe even before they realize it.
Sergey Brin has said he wants to build a benign version of HAL in 2001: A Space Odyssey.This is what intrigues Kurzweil, 65, who has long had a vision of intelligent machines. In high school, he wrote software that enabled a computer to create original music in various classical styles, which he demonstrated in a 1965 appearance on the TV show I’ve Got a Secret. Since then, his inventions have included several firsts—a print-to-speech reading machine, software that could scan and digitize printed text in any font, music synthesizers that could re-create the sound of orchestral instruments, and a speech recognition system with a large vocabulary.
Today, he envisions a “cybernetic friend” that listens in on your phone conversations, reads your e-mail, and tracks your every move—if you let it, of course—so it can tell you things you want to know even before you ask. This isn’t his immediate goal at Google, but it matches that of Google cofounder Sergey Brin, who said in the company’s early days that he wanted to build the equivalent of the sentient computer HAL in 2001: A Space Odyssey—except one that wouldn’t kill people.
For now, Kurzweil aims to help computers understand and even speak in natural language. “My mandate is to give computers enough understanding of natural language to do useful things—do a better job of search, do a better job of answering questions,” he says. Essentially, he hopes to create a more flexible version of IBM’s Watson, which he admires for its ability to understand Jeopardy! queries as quirky as “a long, tiresome speech delivered by a frothy pie topping.” (Watson’s correct answer: “What is a meringue harangue?”)
Kurzweil isn’t focused solely on deep learning, though he says his approach to speech recognition is based on similar theories about how the brain works. He wants to model the actual meaning of words, phrases, and sentences, including ambiguities that usually trip up computers. “I have an idea in mind of a graphical way to represent the semantic meaning of language,” he says.
That in turn will require a more comprehensive way to graph the syntax of sentences. Google is already using this kind of analysis to improve grammar in translations. Natural-language understanding will also require computers to grasp what we humans think of as common-sense meaning. For that, Kurzweil will tap into the Knowledge Graph, Google’s catalogue of some 700 million topics, locations, people, and more, plus billions of relationships among them. It was introduced last year as a way to provide searchers with answers to their queries, not just links.
Finally, Kurzweil plans to apply deep-learning algorithms to help computers deal with the “soft boundaries and ambiguities in language.” If all that sounds daunting, it is. “Natural-language understanding is not a goal that is finished at some point, any more than search,” he says. “That’s not a project I think I’ll ever finish.”
Though Kurzweil’s vision is still years from reality, deep learning is likely to spur other applications beyond speech and image recognition in the nearer term. For one, there’s drug discovery. The surprise victory by Hinton’s group in the Merck contest clearly showed the utility of deep learning in a field where few had expected it to make an impact.
That’s not all. Microsoft’s Peter Lee says there’s promising early research on potential uses of deep learning in machine vision—technologies that use imaging for applications such as industrial inspection and robot guidance. He also envisions personal sensors that deep neural networks could use to predict medical problems. And sensors throughout a city might feed deep-learning systems that could, for instance, predict where traffic jams might occur.
In a field that attempts something as profound as modeling the human brain, it’s inevitable that one technique won’t solve all the challenges. But for now, this one is leading the way in artificial intelligence. “Deep learning,” says Dean, “is a really powerful metaphor for learning about the world.”
人工智慧 挑戰考大學、寫小說
〔編譯林翠儀/綜合報導〕日本經濟新聞報導,為開拓「人工智慧」的無限可能,日本研究人員計畫讓搭載人工智慧的電腦報考東京大學,還要讓電腦在5年後創作4000字的小說。
人工智慧又稱機器智能,通常是指人工製造的系統,經過運算後表現出來的智能。科學家從1950年代著手研究人工智慧,希望創造出具有智能的機器人成為勞動力,但成果仍無法跨越「玩具」領域。
1970年代,人工智慧的研究處於停滯狀態,直到1997年美國IBM電腦「深藍(Deep Blue)」,在一場6局對決的西洋棋賽中擊敗當時的世界棋王。2011年IBM的「華生(Watson)」參加美國益智節目贏得首獎百萬美元,人工智慧的開發再度受到矚目。
日本2010年也曾以一套將棋人工智慧系統打敗職業棋手,國立情報學研究所的研究員更異想天開,打算嘗試讓擁有人工智慧的電腦報考日本第一學府東京大學。
目 前該研究所和開發人工智慧軟體的富士通研究所合作,讓電腦試作大學入學考試的題目。研究人員表示,目前電腦大概能夠回答5到6成的題目,其中最難解的是數 學部分,因為電腦沒辦法像人類一樣,在閱讀問題的敘述文字後,馬上理解題意進行運算。不過,研究人員希望在2016年拿到聯考高分,2021年考上東大。
此外,人工智慧一向被認為「缺乏感性」,因此研究人員還嘗試挑戰用人工智慧寫小說,初步計畫讓電腦寫出長約4000字的科幻小說,並預定5年後參加徵文比賽。
Op-Ed Contributor
What Is Artificial Intelligence?
By RICHARD POWERS
Published: February 5, 2011
Illustrations by Vance Wellenstein
Related
-
Smarter Than You Think: What Is I.B.M.’s Watson? (June 20, 2010)

The question: What is Watson?
I.B.M.’s groundbreaking question-answering system, running on roughly 2,500 parallel processor cores, each able to perform up to 33 billion operations a second, is playing a pair of “Jeopardy!” matches against the show’s top two living players, to be aired on Feb. 14, 15 and 16. Watson is I.B.M.’s latest self-styled Grand Challenge, a follow-up to the 1997 defeat by its computer Deep Blue of Garry Kasparov, the world’s reigning chess champion. (It’s remarkable how much of the digital revolution has been driven by games and entertainment.) Yes, the match is a grandstanding stunt, baldly calculated to capture the public’s imagination. But barring any humiliating stumble by the machine on national television, it should.
Consider the challenge: Watson will have to be ready to identify anything under the sun, answering all manner of coy, sly, slant, esoteric, ambiguous questions ranging from the “Rh factor” of Scarlett’s favorite Butler or the 19th-century painter whose name means “police officer” to the rhyme-time place where Pelé stores his ball or what you get when you cross a typical day in the life of the Beatles with a crazed zombie classic. And he (forgive me) will have to buzz in fast enough and with sufficient confidence to beat Ken Jennings, the holder of the longest unbroken “Jeopardy!” winning streak, and Brad Rutter, an undefeated champion and the game’s biggest money winner. The machine’s one great edge: Watson has no idea that he should be panicking.
Open-domain question answering has long been one of the great holy grails of artificial intelligence. It is considerably harder to formalize than chess. It goes well beyond what search engines like Google do when they comb data for keywords. Google can give you 300,000 page matches for a search of the terms “greyhound,” “origin” and “African country,” which you can then comb through at your leisure to find what you need.
Asked in what African country the greyhound originated, Watson can tell you in a couple of seconds that the authoritative consensus favors Egypt. But to stand a chance of defeating Mr. Jennings and Mr. Rutter, Watson will have to be able to beat them to the buzzer at least half the time and answer with something like 90 percent accuracy.
When I.B.M.’s David Ferrucci and his team of about 20 core researchers began their “Jeopardy!” quest in 2006, their state-of-the-art question-answering system could solve no more than 15 percent of questions from earlier shows. They fed their machine libraries full of documents — books, encyclopedias, dictionaries, thesauri, databases, taxonomies, and even Bibles, movie scripts, novels and plays.
But the real breakthrough came with the extravagant addition of many multiple “expert” analyzers — more than 100 different techniques running concurrently to analyze natural language, appraise sources, propose hypotheses, merge the results and rank the top guesses. Answers, for Watson, are a statistical thing, a matter of frequency and likelihood. If, after a couple of seconds, the countless possibilities produced by the 100-some algorithms converge on a solution whose chances pass Watson’s threshold of confidence, it buzzes in.
This raises the question of whether Watson is really answering questions at all or is just noticing statistical correlations in vast amounts of data. But the mere act of building the machine has been a powerful exploration of just what we mean when we talk about knowing.
Who knows how Mr. Jennings and Mr. Rutter do it — puns cracked, ambiguities resolved, obscurities retrieved, links formed across every domain in creation, all in a few heartbeats. The feats of engineering involved in answering the smallest query about the world are beyond belief. But I.B.M. is betting a fair chunk of its reputation that 2011 will be the year that machines can play along at the game.
Does Watson stand a chance of winning? I would not stake my “Final Jeopardy!” nest egg on it. Not yet. Words are very rascals, and language may still be too slippery for it. But watching films of the machine in sparring matches against lesser human champions, I felt myself choking up at its heroic effort, the size of the undertaking, the centuries of accumulating groundwork, hope and ingenuity that have gone into this next step in the long human drama. I was most moved when the 100-plus parallel algorithms wiped out and the machine came up with some ridiculous answer, calling it out as if it might just be true, its cheerful synthesized voice sounding as vulnerable as that of any bewildered contestant.
It does not matter who will win this $1 million Valentine’s Day contest. We all know who will be champion, eventually. The real showdown is between us and our own future. Information is growing many times faster than anyone’s ability to manage it, and Watson may prove crucial in helping to turn all that noise into knowledge.
Dr. Ferrucci and company plan to sell the system to businesses in need of fast, expert answers drawn from an overwhelming pool of supporting data. The potential client list is endless. A private Watson will cost millions today and requires a room full of hardware. But if what Ray Kurzweil calls the Law of Accelerating Returns keeps holding, before too long, you’ll have an app for that.
Like so many of its precursors, Watson will make us better at some things, worse at others. (Recall Socrates’ warnings about the perils of that most destabilizing technology of all — writing.) Already we rely on Google to deliver to the top of the million-hit list just those pages we are most interested in, and we trust its concealed algorithms with a faith that would be difficult to explain to the smartest computer. Even if we might someday be able to ask some future Watson how fast and how badly we are cooking the earth, and even if it replied (based on the sum of all human knowledge) with 90 percent accuracy, would such an answer convert any of the already convinced or produce the political will we’ll need to survive the reply?
Still, history is the long process of outsourcing human ability in order to leverage more of it. We will concede this trivia game (after a very long run as champions), and find another in which, aided by our compounding prosthetics, we can excel in more powerful and ever more terrifying ways.
Should Watson win next week, the news will be everywhere. We’ll stand in awe of our latest magnificent machine, for a season or two. For a while, we’ll have exactly the gadget we need. Then we’ll get needy again, looking for a newer, stronger, longer lever, for the next larger world to move.
For “Final Jeopardy!”, the category is “Players”: This creature’s three-pound, 100-trillion-connection machine won’t ever stop looking for an answer.
The question: What is a human being?
Richard Powers is the author of the novel “Generosity: An Enhancement.”
訂閱:
文章 (Atom)